Although we think of AI as something groundbreaking, AI’s role in banking and financial services has been transformative since its inception.
23 Nov 2023
Although we think of AI as something groundbreaking, AI’s role in banking and financial services has been transformative since its inception.
The implementation of AI in banking applications and software solutions has significantly revolutionized the way companies access and manage their finances. It reduces costs, increases productivity, and aids in decision-making based on information that would otherwise be incomprehensible to any human being.
Not only that, intelligent algorithms are capable of detecting fraudulent information in a matter of seconds , making AI’s role in banking essential in the fight against fraud.
Already in 2020, Business Insider suggested that nearly 80% of banks were aware of the impact of AI in banking. Three years later, this potential has exploded, and AI is already part of everyday life in the banking sector.
The reason behind this rapid prominence of AI in banking is due to the amount of data that must be used. Banks have all this data, and therefore, are able to use AI to exceed human capabilities and predict outcomes.
When a large number of variables are involved, which is common in this sector, artificial intelligence plays a crucial role, benefiting the business in many ways.
Benefits of AI in banking
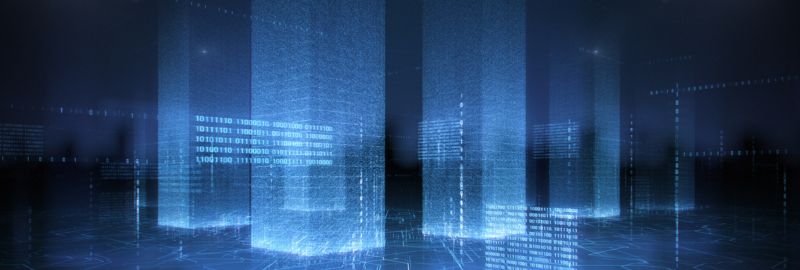
The revolutionary impact of AI in banking is already a fact. Automating processes, improving the customer experience, mitigating risks, boosting efficiency, and transforming overall operations.
However, the applications of AI in banking have not yet reached all their capabilities, and these are just some of the benefits it can bring.
Its future possibilities? They seem, to this day, infinite. As the implementation of AI continues to evolve, it is expected to redefine banking operations in better ways in the coming years.
Improvement in fraud detection
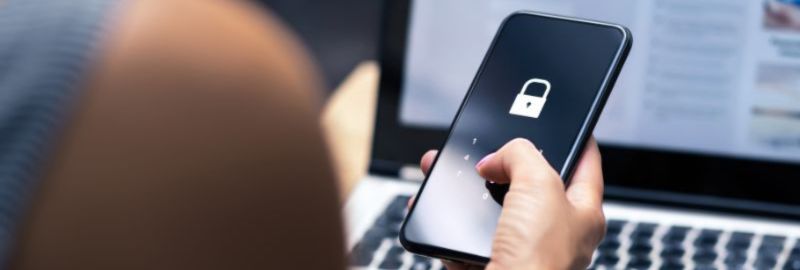
AI can analyze large amounts of data to detect fraudulent transactions more efficiently than humans. Machine learning algorithms can learn from past fraud cases to identify patterns and anomalies that can be used to prevent future fraud.
Millions of transactions occur daily. Users pay bills, withdraw money, deposit checks, and do much more through apps or online accounts. Therefore, there is a growing need for the banking sector to step up its efforts in fraud detection.
This is where artificial intelligence in banking comes into play. AI and machine learning help banks identify fraudulent activities, track faults in their systems, minimize risks, and improve overall online finance security.
AI can also help banks handle cyber threats. In 2019, the financial sector accounted for 29% of all cyber attacks, making it the most attacked industry.
For example, solutions that uses AI like Transmit Security’s detection and response, are a huge help preventing identity fraud, even after a successful phishing attack.
The platform continuously collects information of dozens of parameters, including device fingerprinting, behavioral biometrics, bot detection, network analysis, authentication strength and app activity patterns. Those are cross-correlated with global threat intelligence, fraud patterns and the individual user’s typical behavior, so, as commented, even with the right user credentials theft after a cyberattack, it can be detected and fraud stopped.
This a just an example of how, with the continuous monitoring capabilities of artificial intelligence in financial services, banks can respond to potential cyber attacks before they affect employees, customers, or internal systems.
Personalized customer experiences
AI can help banks provide personalized experiences to their customers.
With the aid of data analysis, banks can understand their customers’ preferences, financial behavior to offer personalized financial solutions. This can lead to greater customer satisfaction and loyalty.
In terms of customer service, chatbots are one of the best examples of practical applications of AI in banking. Once deployed, they work 24/7, allowing humans to use their time more efficiently on inquiries that require personalized attention.
An example of a chatbot for banking applications is American Express and its AmEx chatbot.
American Express credit card holders can link their cards to the AmEx chatbot on Messenger to receive personalized recommendations and notifications.
Contextual recommendations, reminders about exclusive card benefits, etc., are examples of such practices based on its learning.
Operational efficiency
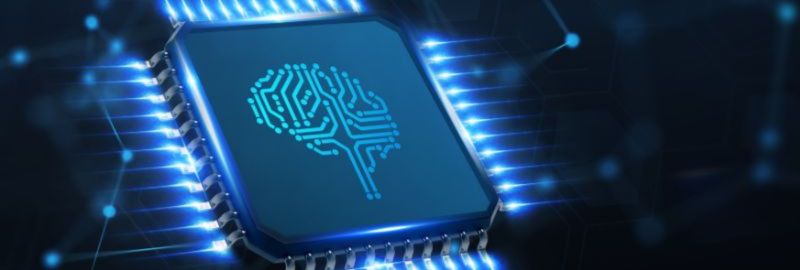
Automation of routine tasks and optimization of operations are two of the key factors in which the role of AI in banking is crucial. Automating processes leads to not only a significant cost savings, but also greater operational efficiency.
With AI in banking, organizations can efficiently automate a wide range of routine tasks such as data entry, account reconciliation, document processing, etc.
It also allows the bank to speed up certain online processes by offering real-time services, since some documents are now not processed manually by humans but by AI, which improves the quality of the final service.
Currently, VASS is using artificial intelligence to improve processes, including generating test cases for code.
This type of automation not only frees up human resources and allows them to focus on more essential tasks, but also reduces the risk of errors and speeds up the completion of processes.
Risk management
External global factors such as currency fluctuations, natural disasters, or political instability severely impact the financial sector. During these times of volatility, it is crucial to make business decisions with great caution.
AI in banking is capable of performing predictive analysis that provides a reasonably clear picture of what is to come, helping the sector to be prepared and to make decisions in a timely manner.
As AI is capable of analyzing large amounts of information, its algorithms can identify patterns of behaviour and transpose them as risk predictors.
This process allows for a reduction in the time and resources needed, as well as offering loans that are safer for both parties.
Undoubtedly, AI is an excellent tool to assist in decision-making, but we must not forget that the final word in all processes should come from the hands of experts.
Risks and challenges of AI in banking
While we have observed numerous advantages of applying AI in banking, as with any technology that advances and changes in a matter of seconds, we must not lose sight of the risks that its use implies.
There are great challenges to be faced in order to use artificial intelligence in a sensible and ethical way.
Data Privacy and Security
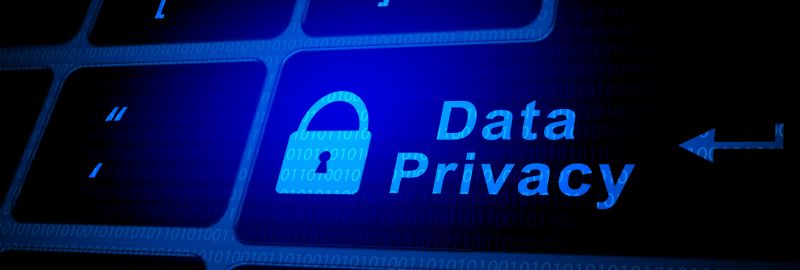
Banks collect large amounts of data from customers, and AI algorithms require access to this data to function effectively.
If sensitive data exist, as is often the case with financial data, any security breach could have serious consequences. Additionally, there is the issue of compliance with privacy regulations, such as the General Data Protection Regulation (GDPR) of the European Union.
In fact, the EU has published a draft law to regulate AI, which details when the artificial intelligence can and cannot be used.
Having specific regulations to oversee the use of AI is vital to ensure data privacy and security, in order to prevent data breaches and protect customers’ confidential information.
Moreover, AI models need to be constantly validated and monitored to ensure they are working as expected.
An example of this is the use of the “HIL”, Human in the Loop technique, which combines humans and machines through the iteration and feedback from both parties.
As AI models don’t always predict with full confidence, humans play a crucial role in providing feedback. They are capable of training and refining the algorithm, improving it over time.
Undoubtedly, it means a great challenge, given the dynamic and complex nature of AI algorithms.
Ethical considerations
The use of AI in banking raises ethical concerns, such as bias in decision making and discrimination.
AI algorithms can be biased if the data they were trained with are biased. This could lead to unfair or discriminatory decisions, such as loan denials based on demographic factors.
Banks must ensure that their AI systems are transparent, fair, and impartial. Alert systems can be used to flag unusual or unexpected actions to employees for further analysis.
As said before, with human in the loop processes, decisions that are made by the AI will be executed only after they have been approved by a human. Both of these systems have limitations, but they are key tools for risk mitigation.
Customer centered work
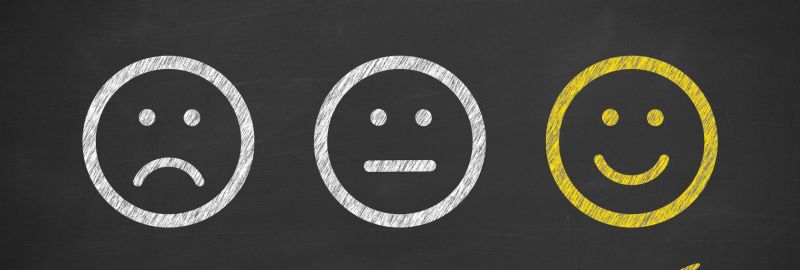
Aside from complying with governmental and sector-specific regulations, banking must always work in the customer’s interest.
For this reason, banking teams must work with a full understanding of how AI and its integration into the customer’s daily life is affecting them.
Not having full knowledge about this factor could put the customer at risk, and the loss of credibility for the entity would be enormous.
Relying solely on a third party, such as AI, leaves companies in situations where they are unable to explain how artificial intelligence models make decisions.
For this reason, risk and compliance teams are especially important in the process of ensuring the reliability of processes that involve the use of AI.
AI’s role in banking presents exciting opportunities, but it also poses significant challenges.
Banks must carefully consider the ethical, regulatory, and security implications of adopting AI to ensure they leverage the technology effectively and responsibly.
In doing so, banks can provide better customer experiences, optimize operations, and manage risk more effectively.
The key to a successful adoption of AI in banking is to find the right balance between innovation and risk management.
VASS is currently working with Machine Learning (ML), Incremental Learning and Natural Language Processing (NLP) to help clients to avoid, among other things, false positives and strengthen their own AML (Anti-Money Laundering) policies.
Natural Language Processing (NLP) permits to analyze and understand the textual information associated with transactions, such as reference notes or descriptions. This can provide more context and help identify deceptive transactions.
Through Incremental Learning, as new transactions are received, the model adapts nearly in real-time to prevent its obsolescence.
Thanks to Machine Learning for Behaviour Modeling, it is possible to analyze transaction history and build behaviour profiles of customers and suppliers. It allows the identification of normal activity patterns and the detection of anomalies that might indicate fraudulent activities or identify false positives.
The artificial intelligence has transformed the banking landscape, offering personalized, efficient, and real-time solutions that enhance the customer experience and optimize internal operations. However, it is not without challenges.
Data privacy management, ethics in automated decision-making, and the potential to perpetuate pre-existing biases are areas that require careful scrutiny and regulation.
Banking, as a crucial industry in people’s daily lives, must address these risks with transparency and responsibility.
Adopting artificial intelligence in banking is not just a matter of technological innovation, but also of trust and ethics. By properly balancing the benefits and risks of AI, the banking sector can lead the way to a more efficient and inclusive future, where humans and machines work together.
Learn how we are helping our clients to leap into their future with AI!